The field of advertising AI is beaming with excitement as the release of ChatGPT, an AI chatbot by OpenAI, impressed people from all kinds of industries and positions with its ability to understand and emulate human conversation.
While there are many possible use cases for ChatGPT and other AI, there are some potential concerns with the methodology to consider. In this post, we cover 5 issues that need to be addressed before AI hits mass adoption.
Quick Recap:
ChatGPT is an AI chatbot, developed by OpenAI, that’s making waves because of how natural and human it replies, as well as other capabilities like generating code, headlines, and even full blog posts. You can check out our previous post on ChatGPT and advertising AI use cases for more details.
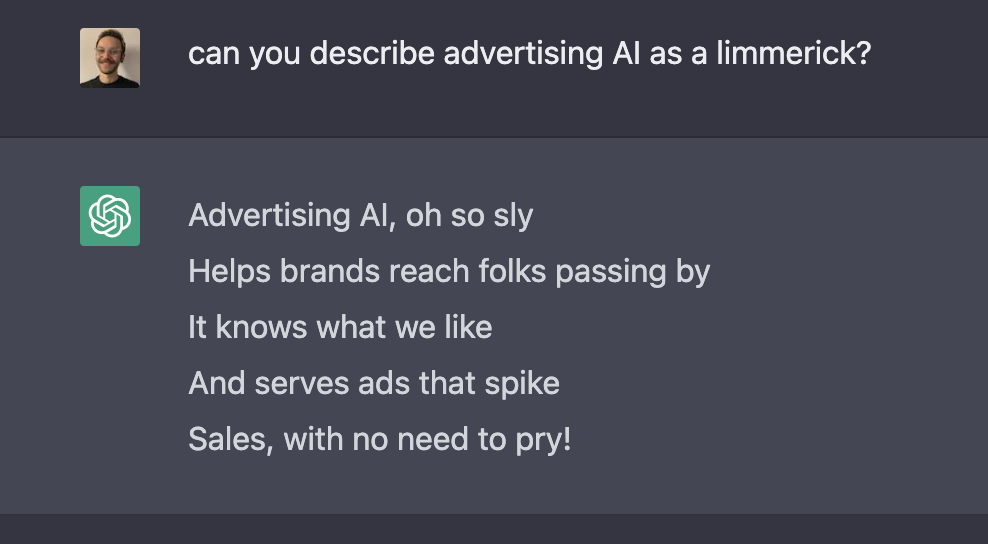
But ChatGPT and other AI aren’t all sunshine and roses. Let’s take a look at some concerns that could affect the future of AI in advertising.
5 Potential Issues with ChatGPT and Advertising AI
1. Data Quality
Training AI is very similar to studying for a test. You have the study materials (AI: data sets), the act of studying (AI: machine learning), and the final test (AI: command output.)
Let’s start with the data sets.
For AI to provide the best possible results, it needs to be trained on the best possible data. You can spend hours studying the wrong things, but you’d still fail the test.
Then, there’s machine learning.
Machine learning is a set of algorithms that AI uses to interpret the data it’s given. You can have the best study materials, but if you don’t study or don’t retain the information, chances are you won’t be able to answer all the questions. For AI, machine learning is evaluated based on accuracy, precision, and efficiency.
Finally, there’s the actual test.
AI needs to be able to interpret the command it’s given and provide an accurate answer. However, this could mean several different things. It could be a chatbot like ChatGPT, an image generator like DALL-E-2, or something behind the scenes like automated tasks. Regardless of what its purpose is, it needs to be able to fulfill that function to nearly the same quality of a human.
2. Data Privacy
As we know, AI requires large amounts of data sets, however, this can sometimes include consumer data. How will AI and its developers respect consumers’ data privacy rights? One potential solution could be to train advertising AI using cohorts or anonymized data, but then how accurate will the responses be?
It’s kind of like trying to study for a history test with all the names, dates and places removed.
Another potential concern is that malicious actors could find a way to inspect the AI’s code and data sets and uncover hidden and private consumer data.
3. Unintentional Bias
Additionally, training AI requires historical data sets, there’s a possibility that AI can perpetuate some ingrained societal biases. A more infamous example of this is when Microsoft released Tay, a Twitter chatbot, which went off the rails after Twitter users were teaching to it be racist and misogynistic.
Since then, developers have been training AI to catch and remove these biases from learned data sets. However, that could also bring about some unintentional biases that arise from correcting old ones.
4. Transparency
Moreso, ChatGPT is what’s known as a “black-box model,” meaning that it doesn’t show or explain the reasoning behind its answers. That can make it difficult to verify how valid the responses are, especially in cases where it could have a significant impact.
For example,
So how can AI become more transparent while remaining private and secure? It’s a delicate balance that developers need to consider with care.
5. Regulation
And lastly, there’s no regulatory oversight for AI models. If something wrong happens, like with any of the potential concerns mentioned above, who’s responsible? Is it the developer or the user?
Additionally, stricter data handling and protection laws and regulations could affect the quality of data that are used to train the AI. Who’s responsible for auditing the data that’s used? These are just some of the many questions that developers, brands and advertisers need to be aware of when implementing AI.
The Future of AI in Advertising
Despite some of the data, transparency and bias concerns, there’s still a bright future for AI. It’s a field that’s still developing and improving daily. With proper and ethical use, AI has the power to not only improve digital marketing campaign performance, but to also make advertisers’ and brands’ lives easier.
Discover how Sharethrough uses AI to improve the performance of digital ad campaigns.
The field of advertising AI is beaming with excitement as the release of ChatGPT, an AI chatbot by OpenAI, impressed people from all kinds of industries and positions with its ability to understand and emulate human conversation.
While there are many possible use cases for ChatGPT and other AI, there are some potential concerns with the methodology to consider. In this post, we cover 5 issues that need to be addressed before AI hits mass adoption.
Quick Recap:
ChatGPT is an AI chatbot, developed by OpenAI, that’s making waves because of how natural and human it replies, as well as other capabilities like generating code, headlines, and even full blog posts. You can check out our previous post on ChatGPT and advertising AI use cases for more details.
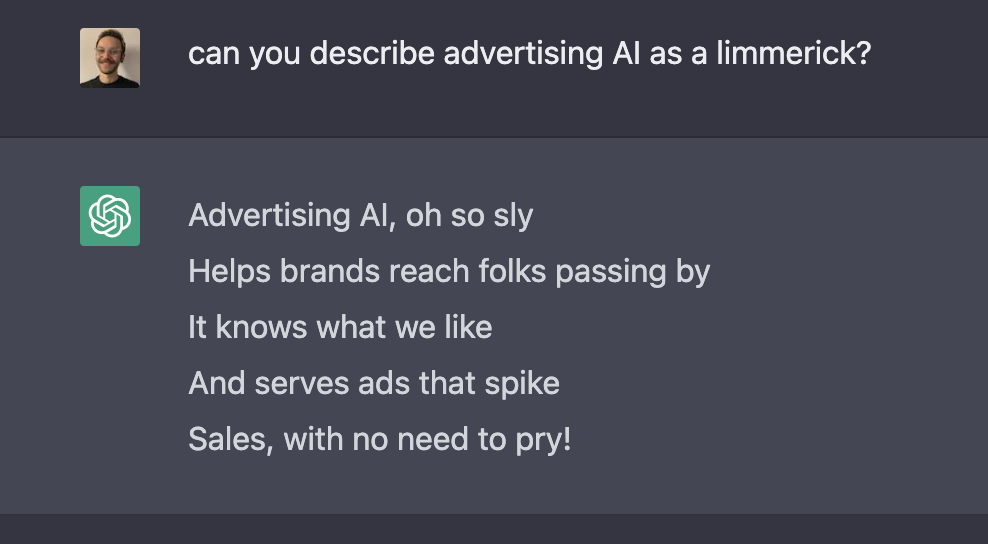
But ChatGPT and other AI aren’t all sunshine and roses. Let’s take a look at some concerns that could affect the future of AI in advertising.
5 Potential Issues with ChatGPT and Advertising AI
1. Data Quality
Training AI is very similar to studying for a test. You have the study materials (AI: data sets), the act of studying (AI: machine learning), and the final test (AI: command output.)
Let’s start with the data sets.
For AI to provide the best possible results, it needs to be trained on the best possible data. You can spend hours studying the wrong things, but you’d still fail the test.
Then, there’s machine learning.
Machine learning is a set of algorithms that AI uses to interpret the data it’s given. You can have the best study materials, but if you don’t study or don’t retain the information, chances are you won’t be able to answer all the questions. For AI, machine learning is evaluated based on accuracy, precision, and efficiency.
Finally, there’s the actual test.
AI needs to be able to interpret the command it’s given and provide an accurate answer. However, this could mean several different things. It could be a chatbot like ChatGPT, an image generator like DALL-E-2, or something behind the scenes like automated tasks. Regardless of what its purpose is, it needs to be able to fulfill that function to nearly the same quality of a human.
2. Data Privacy
As we know, AI requires large amounts of data sets, however, this can sometimes include consumer data. How will AI and its developers respect consumers’ data privacy rights? One potential solution could be to train advertising AI using cohorts or anonymized data, but then how accurate will the responses be?
It’s kind of like trying to study for a history test with all the names, dates and places removed.
Another potential concern is that malicious actors could find a way to inspect the AI’s code and data sets and uncover hidden and private consumer data.
3. Unintentional Bias
Additionally, training AI requires historical data sets, there’s a possibility that AI can perpetuate some ingrained societal biases. A more infamous example of this is when Microsoft released Tay, a Twitter chatbot, which went off the rails after Twitter users were teaching to it be racist and misogynistic.
Since then, developers have been training AI to catch and remove these biases from learned data sets. However, that could also bring about some unintentional biases that arise from correcting old ones.
4. Transparency
Moreso, ChatGPT is what’s known as a “black-box model,” meaning that it doesn’t show or explain the reasoning behind its answers. That can make it difficult to verify how valid the responses are, especially in cases where it could have a significant impact.
For example,
So how can AI become more transparent while remaining private and secure? It’s a delicate balance that developers need to consider with care.
5. Regulation
And lastly, there’s no regulatory oversight for AI models. If something wrong happens, like with any of the potential concerns mentioned above, who’s responsible? Is it the developer or the user?
Additionally, stricter data handling and protection laws and regulations could affect the quality of data that are used to train the AI. Who’s responsible for auditing the data that’s used? These are just some of the many questions that developers, brands and advertisers need to be aware of when implementing AI.
The Future of AI in Advertising
Despite some of the data, transparency and bias concerns, there’s still a bright future for AI. It’s a field that’s still developing and improving daily. With proper and ethical use, AI has the power to not only improve digital marketing campaign performance, but to also make advertisers’ and brands’ lives easier.
Discover how Sharethrough uses AI to improve the performance of digital ad campaigns.
Behind Headlines: 180 Seconds in Ad Tech is a short 3-minute podcast exploring the news in the digital advertising industry. Ad tech is a fast-growing industry with many updates happening daily. As it can be hard for most to keep up with the latest news, the Sharethrough team wanted to create an audio series compiling notable mentions each week.
The field of advertising AI is beaming with excitement as the release of ChatGPT, an AI chatbot by OpenAI, impressed people from all kinds of industries and positions with its ability to understand and emulate human conversation.
While there are many possible use cases for ChatGPT and other AI, there are some potential concerns with the methodology to consider. In this post, we cover 5 issues that need to be addressed before AI hits mass adoption.
Quick Recap:
ChatGPT is an AI chatbot, developed by OpenAI, that’s making waves because of how natural and human it replies, as well as other capabilities like generating code, headlines, and even full blog posts. You can check out our previous post on ChatGPT and advertising AI use cases for more details.
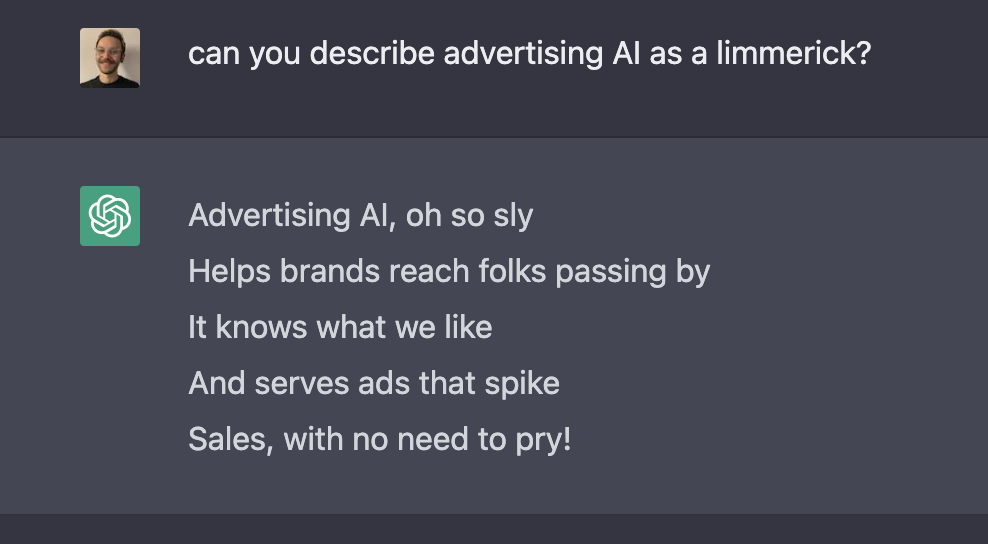
But ChatGPT and other AI aren’t all sunshine and roses. Let’s take a look at some concerns that could affect the future of AI in advertising.
5 Potential Issues with ChatGPT and Advertising AI
1. Data Quality
Training AI is very similar to studying for a test. You have the study materials (AI: data sets), the act of studying (AI: machine learning), and the final test (AI: command output.)
Let’s start with the data sets.
For AI to provide the best possible results, it needs to be trained on the best possible data. You can spend hours studying the wrong things, but you’d still fail the test.
Then, there’s machine learning.
Machine learning is a set of algorithms that AI uses to interpret the data it’s given. You can have the best study materials, but if you don’t study or don’t retain the information, chances are you won’t be able to answer all the questions. For AI, machine learning is evaluated based on accuracy, precision, and efficiency.
Finally, there’s the actual test.
AI needs to be able to interpret the command it’s given and provide an accurate answer. However, this could mean several different things. It could be a chatbot like ChatGPT, an image generator like DALL-E-2, or something behind the scenes like automated tasks. Regardless of what its purpose is, it needs to be able to fulfill that function to nearly the same quality of a human.
2. Data Privacy
As we know, AI requires large amounts of data sets, however, this can sometimes include consumer data. How will AI and its developers respect consumers’ data privacy rights? One potential solution could be to train advertising AI using cohorts or anonymized data, but then how accurate will the responses be?
It’s kind of like trying to study for a history test with all the names, dates and places removed.
Another potential concern is that malicious actors could find a way to inspect the AI’s code and data sets and uncover hidden and private consumer data.
3. Unintentional Bias
Additionally, training AI requires historical data sets, there’s a possibility that AI can perpetuate some ingrained societal biases. A more infamous example of this is when Microsoft released Tay, a Twitter chatbot, which went off the rails after Twitter users were teaching to it be racist and misogynistic.
Since then, developers have been training AI to catch and remove these biases from learned data sets. However, that could also bring about some unintentional biases that arise from correcting old ones.
4. Transparency
Moreso, ChatGPT is what’s known as a “black-box model,” meaning that it doesn’t show or explain the reasoning behind its answers. That can make it difficult to verify how valid the responses are, especially in cases where it could have a significant impact.
For example,
So how can AI become more transparent while remaining private and secure? It’s a delicate balance that developers need to consider with care.
5. Regulation
And lastly, there’s no regulatory oversight for AI models. If something wrong happens, like with any of the potential concerns mentioned above, who’s responsible? Is it the developer or the user?
Additionally, stricter data handling and protection laws and regulations could affect the quality of data that are used to train the AI. Who’s responsible for auditing the data that’s used? These are just some of the many questions that developers, brands and advertisers need to be aware of when implementing AI.
The Future of AI in Advertising
Despite some of the data, transparency and bias concerns, there’s still a bright future for AI. It’s a field that’s still developing and improving daily. With proper and ethical use, AI has the power to not only improve digital marketing campaign performance, but to also make advertisers’ and brands’ lives easier.
Discover how Sharethrough uses AI to improve the performance of digital ad campaigns.
Founded in 2015, Calibrate is a yearly conference for new engineering managers hosted by seasoned engineering managers. The experience level of the speakers ranges from newcomers all the way through senior engineering leaders with over twenty years of experience in the field. Each speaker is greatly concerned about the craft of engineering management. Organized and hosted by Sharethrough, it was conducted yearly in September, from 2015-2019 in San Francisco, California.
Stay Up-to-Date—
Subscribe to our newsletter and receive cutting-edge digital advertising insights, including our weekly Behind Headlines episodes, delivered right to your inbox.